When I speak to clients & colleagues, and read articles here and elsewhere, I come across a common theme - the ideas that people have about price optimisation vary a lot. Whether they have had bad advice in the past, poor implementations that have failed, or have not been given the time to understand it fully, I'm not sure. But there are many basic misconceptions. I've delivered over a dozen price optimisation builds over the last decade, and so here is my attempt to explain what optimisation is and what it isn't.
The Statements
Here's a list of 10 statements about optimisation. Take a moment and write down whether you think each of them is a myth or a fact. We'll deal with the first 5 in this article and the rest in part 2 later this week.
Part 1
- Optimisation is complex, but necessarily complex.
- The primary job of insurers is to predict the cost of claims, not to optimise.
- The 'race to the bottom' for insurance prices was caused by optimisation.
- Optimisation can't predict buying patterns over large premium changes.
- The value from optimisation comes from treating NB and RN differently.
Part 2
- I can't optimise if I send my prices to a broker who can change them.
- Optimisation can work well even in a rapidly changing marketplace.
- If I have larger overheads then I need to set a different optimal price.
- Optimisation can improve your prices across your portfolio.
- It is not possible to optimise in a post GIPP world.
Ok, written them down?
Be honest now, I can tell.
Ok, let's go!
1 - Optimisation is complex, but necessarily complex.
MYTH!
Now this is going to vary depending on what your perception of complexity is, but if we relate it to other parts of working out insurance prices (such as risk modelling) then optimisation is really not that complex. What we do often see is that people have ended up creating some extremely complex rating structures, but these are not only not necessary, but actually harmful.
These problems don't stem from a lack of technical skill, but from a misunderstanding of what is trying to be achieved. If I were to boil down how to improve in this area into 2 statements they would be:
- Pricing is an engineering problem not a mathematical one.
- Think about where you are going not just how you get there.
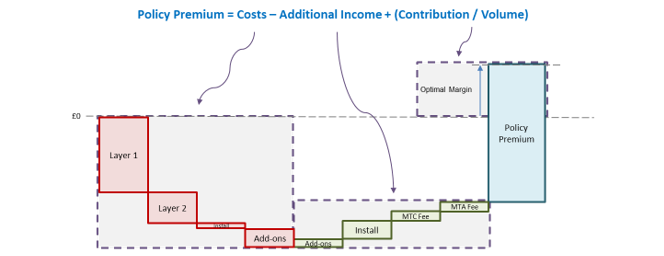
Some companies in an effort to be perfectly accurate to the fraction of a penny end up over-engineering their calculations. Simpler clearer models and structures that are easier to run are also faster to run, meaning that you can optimise more frequently. Think about what every model is adding to you achieving your goals.
The second part really speaks to the need to have one person on your team appointed as the rating structure architect. Their job is to be a specialist in how the rating flows are structured to provide clarity and simplicity, and to design them as a whole, not just step by step. This also increases accountability in your team.
By following these ideals we here at Pebbles have rebuilt optimisation structures that are far simpler and more transparent, but retail a high level of accuracy. Overall they perform better as their is a clearer connection between action and result, and they are also faster to run.
2 - The primary job of insurers is to predict the cost of claims, not to optimise.
FACT!
This statement is completely correct, but it also indicates the strong possibility of one of the most commonly held misconceptions about optimisation. Yes, you should not let optimisation replace accurate risk pricing as the core function of a pricing team - but optimisation is not trying to.
The purpose of optimisation is to take the set of quotes that are closest to the Market Price, and to adjust prices at a granular level to trade them against each other to maximise (optimise) profit (contribution).
And that's it. It should not be replacing your risk pricing because it needs an accurate risk price to assess profit levels. Once you have worked out your technical price (risk + expenses) the role of the retail pricing team is to work out how much profit you can add on top. The role of the optimisation team is to fine tune that profit margin to maximise it. No more, no less.
A modern pricing team must excel at both to succeed. Accept nothing less.
So when we see people say that the primary function of a pricing team is to assess risk price and not to optimise we agree, but we agree because they should not be competing each other, but complimenting each other.

To achieve this we always recommend that you build your rating architecture in a 4 layer structure so that each layer builds upon previous layers:
- Risk - accurately assess the burn cost (Loss Ratio = 100%).
- Expenses - calculate the variable expenses (COR - FE = 100%).
- Retail - work out how much profit you can add (COR < 100%).
- Control - make system adjustments (min prem, etc.).
3 - The 'race to the bottom' for insurance prices was caused by optimisation.
MYTH!
As we just saw, optimisation is the process of trading a few customers here for a few customers there. Putting your price up for a segment of customers that are low value and not price sensitive will result in you losing not many of them and improving margins. At the same time putting down prices for a segment of customers that are high value and price sensitive means you gain a lot of them for a small margin reduction. Combined this means you can offset the losses with gains, and swap low value quotes for high value quotes. This results in an overall improvement in contribution.
This is how "old school" pricing used to be done when I started my career.
Optimisation is no different to this old school segmented pricing - the only thing that has changed is the granularity of the calculation. Rather than looking at the performance of entire segments and lumping changes together as a whole we build models to assess it at an individual level. That's it. That's literally it.
The race to the bottom, where insurers make a loss on new customers to gain sales that then make back their profit margins when they renew, is a fundamental mechanic that exists for 4 reasons:
- Existing customers are ones you know you were price competitive for, so it makes sense you could increase their price and still be competitive.
- Customers tend to stick with what they know unless you upset them.
- Renewing customers tend to have fewer claims so even at the same price they drive more profit.
- If you sell via PCWs you don't have to pay a fee when customers renew.
This is all true whether you optimise or not.
The innovation that accelerated this process was when insurance companies started to combine the value of customers through their lifetime with them. The LTV metric (life time value // long term value) looked at the entire profitability of a customer and made insurers realise that they could maximise renewal profits by discounting at new business.
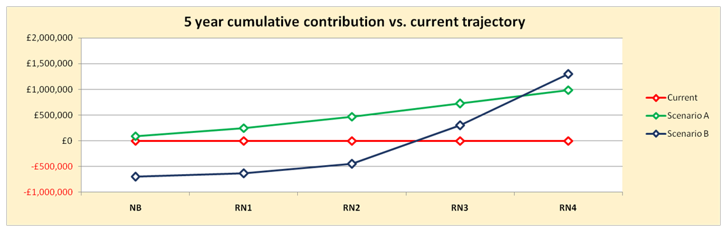
And that's still true today in a post-GIPP world (a recent regulation in the UK that means that customers who are renewing cannot be charged more than if they were a new customer, with a few exceptions like where a telematics score is present). What has happened is that it has stopped the extreme practices which heavily punished loyal customers, and the extremely risky practice where insurers had to make a big loss in Year 1 in the gamble that the person stuck with them long enough. Insurance should be the opposite of gambling.
4 - Optimisation can't predict buying patterns over large premium changes.
FACT!
The best way to predict whether customers will change their purchasing behaviour based on a price change is to actually change their price and then measure it. This is the basis of the price test that many insurers put into their rating, where a small and random number of customers are priced differently.
It is important to understand that this comes at a cost. If your "optimal" price is £500 then allocating a price of £450 or £550 is sub-optimal. Commercially it hurts. But the pain is overcome by the benefit of having that information that you can build into your elasticity models to inform your future price changes.
The issue is that the relationship is not linear. The sales reduction that you see from increasing rates by 30% does not inform what would happen to a 3% increase or a 10%; it just won't be one tenth / third of the effect.
Similarly a price test of +/- 5% can't be confidently extrapolated to 30%. This is because conversion rate vs distance from a competitive price has an S-shaped curve, not a linear one. At some point that price change will move so far away from the Market Price that there will be no impact on conversion rate.
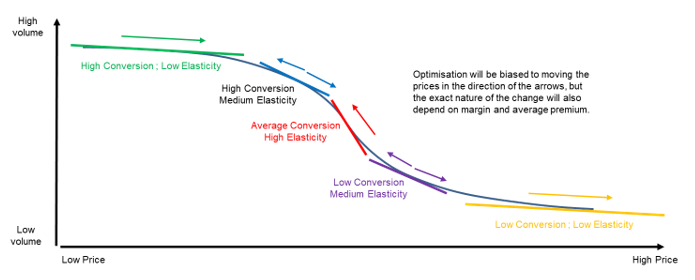
As the majority of your price changes should be small (your risk premiums and other models are based on your current prices, so any large movement will put them at risk of being inaccurate) then your optimisation search space width should also be small. We recommend no more than about x1.5; so if your PT is +/- 5% then your search space should never be more than +/- 7.5%.
So how do you move your prices to the really big changes? Well, this is where you need to change your methodology so that you are optimising on an iterative rather than recycled basis. So instead of using your technical premium as your base for optimisation every time, you use your last set of optimised rates as your base for the next round of optimisation. That way your models have all had time to refresh and update and you build and build on previous successes as your prices iterate towards that optimal position.
5 - The value from optimisation comes from treating NB and RN differently.
MYTH!
As we spoke about before, optimisation is about trading some customers for other customers to maximise overall profit. And whilst having the ability to optimise NB and RN differently does allow for more freedom, it doesn't actually add any value. The value in treating NB and RN differently is explained in Statement 3. The reduction in value from GIPP is about the same whether you previously optimised separately or together.
And whilst many companies did have separate NB and RN optimisation processes, the most successful ones did align them strategically. The best way to get value was to optimise your RN customers first so that you knew what a profitable customer looked like, and then you optimised your NB rates to bring in more of those customers. So whilst the pricing technically was separate they were trying to do the same thing.
That's not possible now, and I won't get into it here, but it is a very good thing for everyone that it is no longer possible. But as we saw LTV is still a valuable metric. Renewing customers still don't have to pay an acquisition fee and still tend to make fewer claims so there is still value in assessing (and therefore optimising) your rates over the long term.
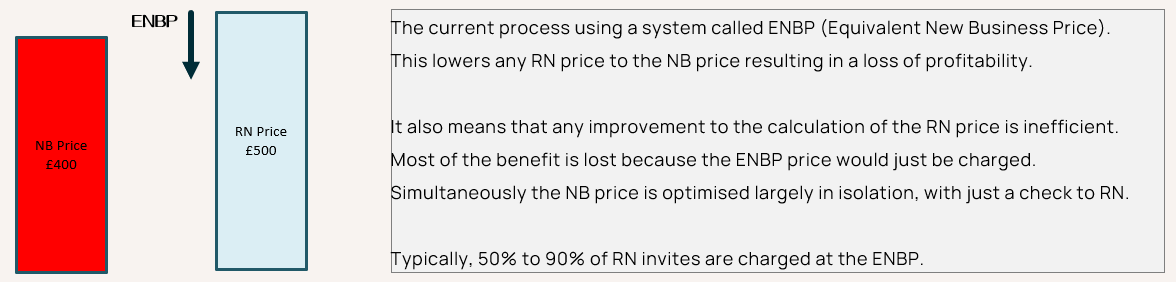
The issue, technically, is how to do this. NB and RN prices have been separate for so long that the industry, almost entirely, adopted exactly the same practice to meet GIPP requirements - run all your RN invites through your NB engine and take the lower of the two. But this does cause problems when it comes to NB optimisation because the impact of the price change to RN customers is not inherent in your calculation. And it causes even more problems to RN optimisation because many (most?!) of your prices won't change as they are capped by the NB price.
This is why we at Pebbles have created Co-Optimisation.
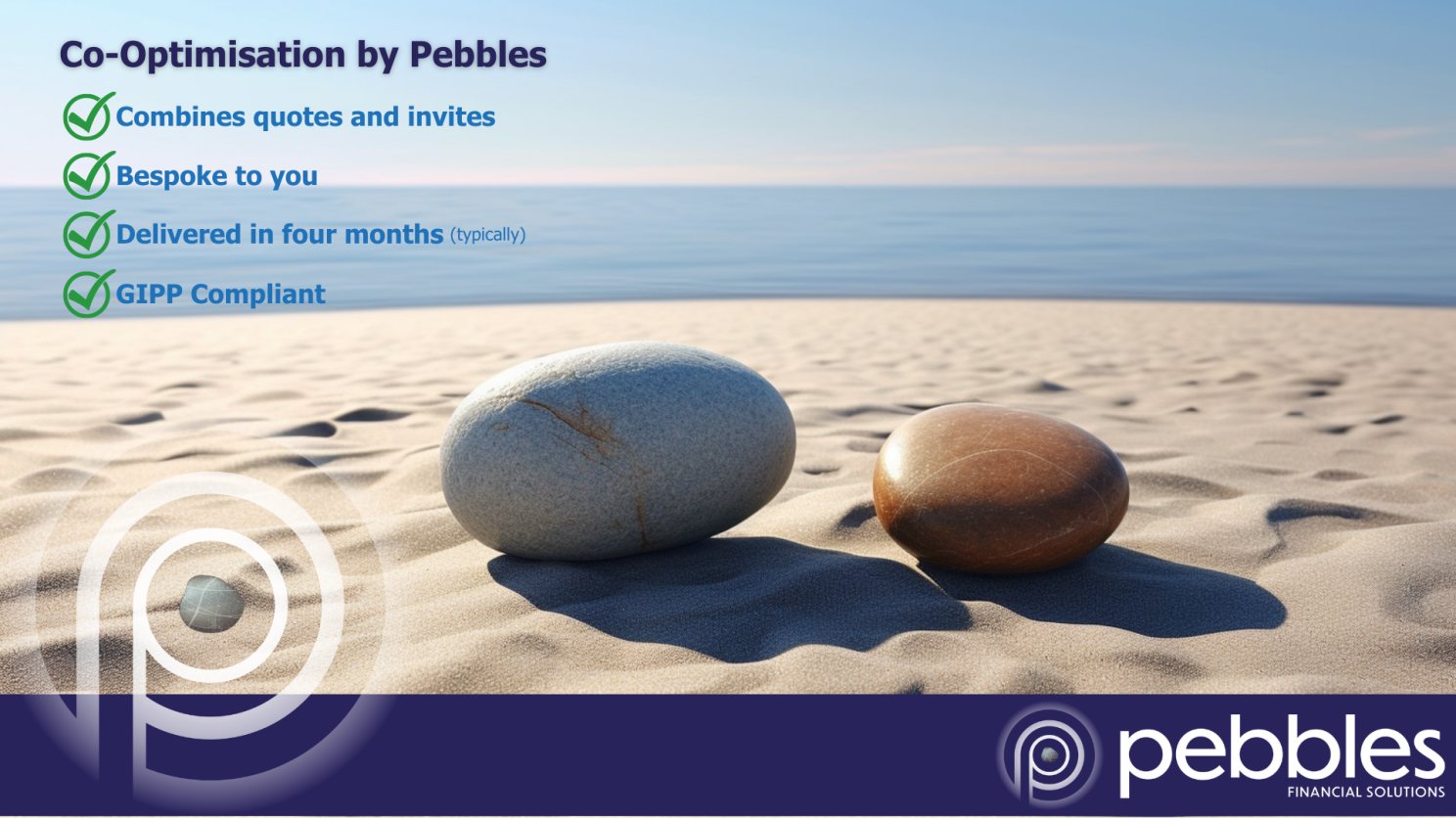
We can combine your ratebooks (on whatever platform you use) so that NB and RN are priced, and optimised, together. It creates a single optimal price for both NB and RN customers that complies with both the letter and the spirit of the GIPP regulation. It doesn't replace the value lost from being able to price walk at renewals, but it's not supposed to and it is not trying to. But what it can do is take into account the impact to both new and existing customers simultaneously.
If you want to have a more in depth discussion about any of the points raised here then please get in contact at Dan@pebblesfs.com or leave a comment on the post. I'm especially interested in the experiences that people have had with optimisation and how it works in your own territories.